Le Show; 2017-11-27
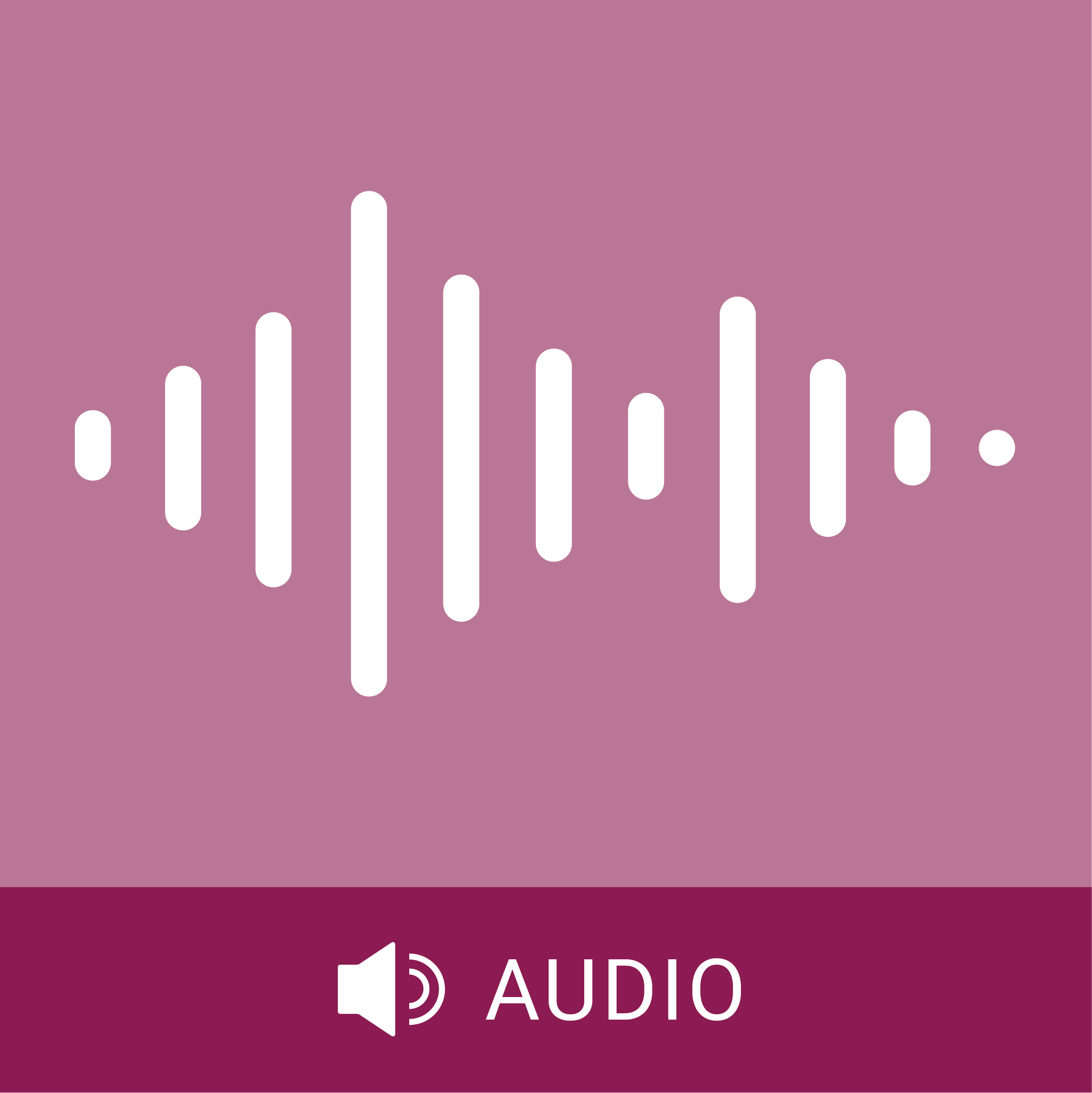
- Transcript
From deep inside your audio device of choice and from so long ago this program was recorded way back last Wednesday the Wednesday before you're hearing it so it's sort of catch-up day and then major information day on the program yeah now I'm naming the days like you do and first off the apologies of the week well given the fact that this is only midweek this this program is being recorded I'm going to feature two apologies you're you're hear them you'll hear the tone of them both both on the subject of sexual misbehavior by powerful men first from Charlie Rose in my 44 45 years in journalism I have prided myself on being an advocate for the careers of the women with whom I've worked nevertheless in the past few days claims have been made about my behavior towards some former female
colleagues it is essential that these women know I hear them and that I deeply apologize for my inappropriate behavior I'm greatly embarrassed I behaved insensitively at times and I accept responsibility for that although I do not believe that all of these allegations are accurate I always felt that I was pursuing shared feelings even though I now realize I was mistaken unquote the incident's involved among other things having female employees at his house when he would walk in from the shower naked or with an open bathrobe as you please this from John Lasseter head of Pixar I've always wanted our animation studios to be places where creators can explore their vision with the support and collaboration of other gifted animators and storytellers as a leader it's my responsibility to be sure that no members of the team failed to feel valued and I now believe I've been falling short in this regard I've recently had
a number of difficult conversations that have been very painful for me it's never easy to face your missteps but it's the only way to learn from them as a result I've been giving a lot of thought to the leader I am today compared to the mentor advocate and champion I want to be it's been brought to my attention that I've made some of you feel disrespected or uncomfortable that was never my intent collectively collectively you mean the world to me and I deeply apologize if I have let you down I especially want to apologize to anyone who has ever been on the receiving ended of an unwanted hug or any other gesture they felt crossed the line in any way shape or form no matter how benign my intent everyone has the right to set their own boundaries and have them respected unquote John Lassiter taking a six month leave of absence from Pixar for what variety described among other things as his tendency to be a quote prolific hugger unquote the apologies of the week later gentlemen a copyrighted feature of this project we are in case you haven't
noticed ladies and gentlemen we are living in a society ruled among other things right now by algorithms and we hear that word all the time some of us toss it off as if we know what it means but the rule of algorithms is getting more and more interesting by which I mean frightening so I have invited to the program today to talk about her book weapons of math destruction Kathy O'Neal it should be doctor isn't shouldn't it really yeah yeah Dr. Kathy you can call me okay it hurts right here Dr. Kathy O'Neal who did undergraduate work at UC Berkeley where you I guess learned to be a troublemaker then did a doctorate in math at Harvard Harvard and then all the positions of the math departments of MIT and Barnard doing research in arithmetic algebraic geometry you had me until the geometry part she worked for four years in the finance industry as a quant
and bailed out of that and has now been writing books on the subject of data and data science welcome thank you Harry I'm so happy to be here so let's let's start with that a little bit of that background when I was learning not to invest in stocks I learned that there were like three main ways to analyze stock buying one was well there's also there's just blind picking like the racetrack but there's technical analysis where people look at charts and somehow figure out that chart movements are predictive value investing sort of the born buffet style where you just I like this company I like the I like their product I like the way they do business I'm buying in for the long term or arbitrage where you're trying to take advantage of momentary or are very brief price differences and and maximize those to your benefit now when quants came in quantitative analysts are they just they just burst a huge hole through arbitrage style analysis is that the
point of the exercise hmm I don't think so now I think it'd be closer to charts I don't think it's perfectly mapping what I did perfectly maps onto that picture but I think it'd be closest to charts what we did was we used historical data going back to like 1980 if we could get it to try to figure out consistent patterns and and you know find statistical patterns that remain consistent and that we could bet on and we would like try to build models that would have made money in the 80s would have made money the 90s and then we would run those models once they were complete on the years after 2000 to see if they would still have worked in the years leading up to the present and if they seem to be money collectors that entire time then we would put them into production and try to make money now so they were looking they were very backwards looking in that in that sense like as almost all algorithms are they were saying whatever happened in the past will continue to happen and the only problem with that is what well sometimes things change I mean
you know once the crisis happened one of the reasons by the way one of the reasons we sort of stopped we sort of didn't test our data on post 2000 until the very end well because first of all it's because it's a good it's sort of a clean statistical methodology that you shouldn't overfit your models but also because it was a real test because after 2001 there was a there was a bubble breaking you know in 2001 and the question the big question was will this model that you've built adapt to post um dot com bubble um environments so it wasn't like we are completely unaware of the fact that climates change um but having said that like the ones that were in production at the at the time of the crisis often did not adapt to the financial crisis because things changed in a different way than they had changed at the dot com bubble moment so yeah I mean anything not only changed but they change in different ways they change in different and fundamentally different ways yeah that's important and was that what drove you out of being a well-remunerated
quant in the financial industry no it wasn't a discovery that I wouldn't make money it was the discovery that I would make money that made me leave it was really a disillusionment I mean I am an idealist I'm actually a hippie as you would guess from my from my Berkeley experience um and I wanted to actually like make the world a better place and I was just incredibly naive when I started finding working in finance in early 2007 about what that would look like so when the crisis you know when the causes of the crisis emerged I saw as planned as day that mathematicians had had a large part in them namely the the AAA ratings on mortgage back securities it wasn't what I was doing exactly but it was close enough for me to be ashamed and yet the people who rent the ratings agencies weren't ashamed well I think they were but that doesn't mean they stopped doing it they weren't ashamed enough yeah so now let's move to the a definitional moment what is an algorithm an algorithm is something that we all do every day
in our heads where we use sort of past information and data to predict future success um so we use data that we've collected and models that we've collected over the years to decide for example like what to major in in college if I majored in this then this is what would happen to my life if I majored in that that's what would happen in my life so we we are using information that we've collected not about ourselves but about other people and we're sort of adapting it to our situation saying will I be successful if I do this um and so just with that very generic definition I would argue that we do it all all the time we don't we almost never formalize it in computer code that's what my job was is to formalize models in computer code and you know we don't necessarily do it in important ways most algorithms are completely unimportant in fact but there are some algorithms that are very very important indeed I think the most important thing to know about algorithms is that they're not inherently fair they're not inherently
neutral or objective that we put in a lot we we sort of project a lot of our agendas onto the algorithms as we build them at the very least because we define success I mean and we I would define success in a different way than someone else might define success you define it the way happy defines success right yeah exactly I would define success as like the world is at peace and we know how to share and that's not that was not you know a shared definition of success most people if they have the right incentives in place would want to build accurate algorithms but when you have the wrong incentives in place you actually benefit from wrong being wrong so that's exactly what happened with the triplet ratings like the people building those risk models had plenty of reasons to not trust them but they benefited from from lying um and you know so when people talk about algorithms here's what I want you to keep an eye out for they're gonna they're gonna use examples like chess or go or sports which are all fun things to think about I love those things but they have a very clear definition of success that everyone
has agreed upon and that's a big big difference with the kind of algorithms that I worry about like things about like who deserves an insurance who deserves a credit card is this a good public schoolteacher does this criminal defendant look scary and should they go to prison for longer than others um those are those are questions where the definition of success is certainly not well understood and the different stakeholders in the situation all have just you know disagreement on what success looks like in the book uh weapons of math destruction you uh go through several of those uh kinds of decision matrixes that you were just talking about and um um you compare decisions based on these models these algorithms with the old days where let's say a banker is a community banker is sitting there at behind a desk and a member of the community comes in for a loan applying for a loan and uh the banker applies a certain objective criteria how much do they make uh they afford the payments and then a host of subjective criteria which we all have
do I like these kinds of people houses person dressed do they fill out the forum using proper grammar and is there handwriting sloppy or not and moving to algorithmically based uh an automated decision making supposedly makes it more objective that's the theory right well that's the marketing yeah I wouldn't even call it a theory because it's not it doesn't rise to level theory but that's definitely the marketing the marketing is that if you use you know big data algorithms you're going to get a lot more information that's relevant um and then it's going to be neutral it's not going to have an agenda you're not going to have human bias human bias will be somehow be um cleared away and yet well there's lots of problems with that and I think the number one is that there's no such thing as human bias being cleared away um for two reasons um at least the first one is the the data that we've collected is a historical artifact of our society so um one thing that you know a credit company might do because they need to use data to train their algorithms is
to use historical data they just say okay historically speaking who was more likely to pay back their loans in the past um and depending on how far you go back um the answer is definitely going to be white men um because of the way that other people were prevented from getting good employment for example and so if they just follow the data as it were they would say oh well then obviously we should fairly objectively and neutrally only give um credit to white men um and that's just I mean it's an extreme example and it's it's a comically extreme it's not that simple um but that's just to say that data itself is not an objective observer it is collected by humans uh and it is reflection of our society it's a reflection of the society that it is surveilling um another example I like to give is I use an algorithm inside my head every day to cook dinner for my kids and the data I use are the ingredients of my kitchen which I'm I've already lied because I don't use all the ingredients in my kitchen my teenagers have talked me into keeping a supply of
ramen noodles those in those little plastic packages but I don't really think they're in food so I never use them to cook dinner so I I am already sort of carving out an exception like I'm carving up the the data that I decide is relevant and that's my bias that's my subjective choice and then when I'm done with feeding my family I decide whether the dinner was a success which I defined because I'm the one in power and my definition of success is if my kids ate vegetables it was a good meal and of course you know my youngest son who loves Nutella he has a different definition of success and it matters over time I optimize to success so I I'm much more likely to make a meal that was what I thought was successful in the past or a meal similar to a meal that was successful in the past and so the sort of arc of meals in my family in my family home is you know very much related to my definition of success so that's the other thing about bias is that we define success so credit is usually pretty straightforward credit is like do you pay back your loan but think
about something a little bit less straightforward like should I hire someone like you well should I hire someone like you first of all there's all sorts of historical bias about if so if we only go by who did I hire in the past that's already a problem but then there's also a very subjective definition of success like how do I know if somebody was successful or will be successful and again if I look at historical data I might I might choose to define success by someone who was who who was promoted a lot and who was given a lot of raises in the past so people who in the past were given lots of raises who were promoted a lot who stayed for a long time but if you think about it like it's the culture that decides who deserves a promotion it's a culture of a company that deserves who gets you know a raise and it for that matter you know the culture decides who feels comfortable to stay for a long time so when you when you optimize to that kind of success you're blinding yourself to the cultural effects of what kind of what kind of effects there are
internal to your company that might make someone look successful or look unsuccessful that's the kind of thing I really worry about because this is what algorithms are being used for nowadays all sorts of difficult messy decisions that people don't really want to think too hard about because it does get complicated and so they're sort of handing it over to the machine and saying hey give us give us sort of sanitized scoring system that we can point at and call it scientific it's not at all scientific it's just it is sanitized it's it's but it's embedded all of our historical mistakes as well as successes and we haven't come to terms with that so previous advantage is is being embedded into the assumptions of the system or it's being blind to the fact of previous advancing in looking at the historical record yeah I mean I sometimes even extreme thought experiment with Fox News Fox News is it is it an extreme thought experiment yeah yeah so imagine
like Fox News decides to build a hiring algorithm and so they have all this data which is just the 21 years of people trying to apply for jobs at Fox News some of them get that offer so that's a signal some of them stay and some of them get promoted or or they get raises those those are all signals but for the sake of the thought experiment we can make the assumption that women even qualified hardworking women were systematically denied success they were systematically denied promotions and raises they were made to feel uncomfortable so they left early so if we simply define success as someone who you know gets lots of promotions and raises and stays for a long time and then we ask the and we train our algorithm to find find success which is what we do this is how we train algorithms then when you apply that algorithm to the current pool of applicants for Fox News what will happen well what will happen will will be that the the women will be filtered out
systematically they will be filtered out because they do not look like the people that were successful in the past and that's sort of that's the sort of deep point about machine learning all these algorithms is that they do not make things fair they simply propagate the the status quo they automate the status quo which is to say like if we had a perfect company with a perfect hiring system and perfect ways of deciding who deserves a raise and who goes deserves promotions and a welcoming atmosphere like if we had all that then we would probably want to automate it because we'd be like oh dude we did all this work let's make this official let's formalize this in code but we don't have that I mean Fox News is an extreme example as I said but it's not that extreme actually most companies have plenty of implicit bias so if we wanted to evolve past what we've been doing in the past like if we want to actually get better then we would do the opposite
of of formalizing it and automating it we would look at ourselves we would examine our practices and say how can we do better and that's the opposite of what we're doing with big data let's take something that's not a thought experiment that that you point out in your book which is gone from being kind of a nice marketing exercise for a failing weekly news magazine to something that affects the decisions and the fates of hundreds of thousands of young Americans and the people who make decisions at colleges and universities that's the US news college ratings talk a little bit better how this what we've been speaking about is embedded in that in that rating system right so I mean and it's a great example of what I call the feedback loop at the society level that these algorithms don't just don't just exert power on the on their targets they really they create all these other sort of feedback loops and pressures on society and I
really think the US News and World Report college ranking model is just the perfect example of that and I should add that it's not big data at all it's actually small data but what they did which was very advanced in the sense of like they were early on it was that they sort of marketed it as objective and fair and neutral and the way they did that was they just kept it secret on the one hand but on the other hand they call it they called it quality and I think the word quality like deserves a medal in the sense that like it means nothing but it it sort of creates trust in the people that hear it so people just like oh well what's the highest quality college and they just loved loved these lists people just love lists but in this case what happened was a sort of magazine who which was going out of business probably decided to fry this out and it was a huge success it wasn't based on particularly good data a lot of it was self-reported and yet people just
loved it and so as soon as parents started really loving it that meant that college has started paying attention and then as soon as colleges started really paying attention so they started gaming it which is to say they tried to up their rank by any means necessary so they had to kind of backward engineer what what was going into the ranking system what were the data ingredients and actually since it was self-reported in a large part like they got to know that pretty well so for example they knew that the average score of their incoming freshman class of average SAT score wasn't was something they knew that the number of kids who applied but didn't get in was important they needed to look exclusive and they started just doing stuff that would make them look better make them look more exclusive make their kids look smarter make their kids happier so they can get fancier kids and I'll say one thing that the the college ranking system didn't care about
and this is just as important as what it does care about is it didn't care about cost you just simply did not take into account cost which you know like going back to the idea of like how much we love lists I think if we were if anybody had been asked if I sort of average parent in in America that I asked like what do you care about when it comes to your kids college cost would have been up there with you know in the top five let's say it that way but this was blind to cost and the result of it being blind to cost would meant that the college administrators who spent a lot of time and effort to to game the system could also spend a lot of money to game the system and it would and the tuition would rise but the model would not care and for them it just again by any means necessary and sometimes you saw pure gaming sometimes you saw out now cheating you see cheating still and the as the college rankings generalized to law school rankings etc you saw cheating by the law schools as well you just saw a bad self reporting like just lies you saw manipulation it was
a it was a real mess and it and it continues to be a mess and the most I guess the the saddest thing about it to me is that like it also made the experience of being a high school kid worse because some of the lengths they went to to make themselves look exclusive were we're actually sadistic I mean you have some colleges that would get kids to apply even though there was no chance they'd ever get in just because it would sort of boost their exclusivity metric it also there's reason to think that some schools made it harder to harder for good kids to get in because they didn't like the idea that they're they were a safety school because that was another metric that the college ranking cared about like the number of kids who actually come that you admit so there's all sorts of ways in which kids experience applying to college has gotten worse indirect reaction to the college ranking I have to say I find it hard to believe that anybody could make the experience of being a high school kid worse but I know onward to education
as it's practiced in the sub university level you go back to a report that made huge waves that was issued during the Reagan administration called a nation at risk which sort of set the agenda for the debate that is still ongoing about public education in the United States talk a little about that yeah I mean nation at risk basically panicked people and started the sort of teacher accountability movement and it was a kind of a sad sort of mathematical mistake actually so basically it reported that SAT scores were going down on average and so technically they were but it was actually happening under the covers and they were looking at like I think 40 year span from like 1960 to you know maybe maybe 20 years like 1960 and 1980 and what was really happening was way more kids poor kids were going to college way more poor kids were going to college
and their scores were lower than rich kids so if you think about it in 1960 it was a pretty elite thing to go to college by 1980 like it was considered a thing you you strive for in the middle class and since the scores for poor kids were lower the average actually went down but the but the reason it's a mathematical mistake actually has a name called the Simpsons Paradox is that in the same 20 in that same 20 year period if you had only looked at poor kids their scores went up if you'd only looked at rich kids their scores had gone up so every category of kids actually got better at the test but the average went down simply because the makeup of who was taking the test had changed in those 20 years so it looked like bad news but if you sort of dug down in the data a little bit it was actually good news in in lots of ways like more kids going to college everyone doing better on the test etc but yet it was it was taken the wrong way and kind of you know deliberate sense
and it sort of spurred on I get as I said the teacher accountability movement where we had you know since then essentially and maybe until Trump we had every every president wanting to be the the president who fixed education and usually what that could be translated in two different directions one could be international competitiveness which is one thing and then the other thing which is usually taking a little more seriously within the states is this idea that we have to close the achievement gap so we have to close the difference between average test scores of rich kids and poor kids and as I said before there is a gap and here's another thing it's actually growing because although as I said poor kids are doing better on tests rich kids are doing better more they're faster so the gap is growing and the idea was okay we're going to close this gap and the way we're going to do that is we're going to get rid of the bad teachers and the bad teachers must be the problem here and so there's been just a sort of war on teachers ever since
and how have algorithms or weapons of math destruction in your usage played into that well the first thing is I guess the first and last thing is that you have to find the bad teachers if you're going to get rid of them and we find the bad teachers with scoring systems basically and the the first generation of scoring systems was really stupid it was just simply like to find a teacher to be bad if a like a lot of their their students don't do well in test and as I just said you know poor kids are much less good at tests the rich kids so when you say that when you you're just putting a sort of target on the back of teachers of poor children so that was the first generation of sort of teacher accountability it was obviously unfair to the teachers of poor kids especially in inner cities so they came up with a new method called the value-added teacher model which was to be fair to them like an effort to make this a fairer system
but the problem was that it was just statistically quite random so I found a teacher who got a six out of 101 year and then next year he got a 96 out of 100 and he figured out how that had happened so a little bit about the value-added model the idea with the value-added model again it's based solely on test scores so if you're looking for some kind of deep understanding of pedagogy you don't look here it is simply like are these kids doing well on tests but in this case wasn't are they doing well relative to some abstract benchmark in this case it was are they doing well compared to what they were expected to do so yeah so that means that each of them has to have an assigned expected score and that is the algorithm what is your expected score and the problem is that it's really hard to guess what a kid's going to get so these expected scores had lots of uncertainty attached to them and then the teacher in question would be assessed based on the
difference between the scores the kids actually got versus the scores they were expected to get so if like little Johnny was supposed to get a 75 but he only got a 70 the teacher would be sort of dinged for five points like teacher did not live up to expectation for Johnny but if Mary was supposed to get a 75 and she actually got an 80 then the teacher would be sort of given credit oh you did better than expected for Mary and so does that make sense so you're like a bump down for Johnny a bump up for Mary and you know keep going and if you have 30 kids then you have 30 bumps some of them are up some of them are down and the teacher gets sort of the average bump that's there that's how they get graded the problem of course as I already suggested is that there's lots of uncertainty both for the expected score for Mary and Johnny and all the other kids as well as the actual score because if you think about it like on a given test day you might have missed breakfast or you might not have slept well or you know it might be hot that day but you don't have air conditioning or the test itself might be easier that year because it's the election
year you know there are all sorts of reasons that those numbers are uncertain and that difference between actual and expected is called the noise term in the original expected expected score model the teacher has 2530 kids so they're being they're being assessed based on the average of 2530 noise terms which sounded like a very statistically very bad model to me and I tried to get sort of the formula but I couldn't get it and and so it it looked like a lost cause but then but then at least for the New York City model the value item model for New York City teachers the New York Post had actually gone to the trouble of getting the names and scores of all the teachers and and sort of publishing them as a sort of active teacher shaming for the worst scoring teachers and then this New York this New York City high school teacher Gary Rubenstein at Stiviston High School he got his his hands on that data and he found more than 600 teachers that actually had two scores for the same subject for the same year and he plotted them and they looked almost completely
random so it was like I think it's fair to say that this was almost a random number generator so teachers were getting almost random numbers as their overall score and yet we still have the situation where if you got a bad score for a couple years in a row you could be denied 10 year and in some places like Washington DC and Houston you could also be fired for bad scores and people were fired which was just an outrage so we have what we have is like an important white spread algorithm secret to everyone including the Department of Education officials who are using it which is nevertheless sort of assigning random numbers to people and some of them are getting fired for it and the teacher we mentioned earlier mr. Clifford had figured out why he scored a six one year in a 90 something the next year which was what I believe what happened was when he scored the six he had kids that were very high performing and it's really really hard if your kids are averaging 98 99 points you know percentile to get them higher like how can you get
go above expected if they're already in a plus I mean you're just you're just hitting against that limit of 100 percent so he thinks that you know just because a couple of them got 95 he looked terrible and then with the 96 he had another classroom where they had a real potential and they met their potential but his point is it like he didn't change the way he taught he didn't change himself as a teacher and this is a guy who had 20 I think 26 years experience teaching English you know he wasn't gonna change and he didn't he his tenure wasn't on the line but his point to me was that we have all these other teachers that were young they were new and they were incredibly destabilized and demoralized by this arbitrary system of punishment and it really was arbitrary I mean there's reasons to think that I want to stay in the in the world of education to talk about for-profit colleges but we have to get to jobs and credit first maybe we can circle back to for-profit colleges but credit scores and e scores take us through that a little bit
if you press right so credit scores people don't like them and I don't like them either because they're used poorly but I'm going to give some credit to credit scores which is that there are rules for credit scores and there should be more rules but one of the great things about credit scores is that there's a series of of laws I think from the 70s one of them was called the Equal Credit Opportunity Act and that meant that you couldn't use race or gender to decide people's credit score or their creditworthiness and the other one is the Fair Credit Reporting Act which said that you should have access to the data going into your credit score and you should be able to complain if the data's wrong and that's where you get like the free credit reports that's why we can have we can see our free credit reports so now like fast forward to you know the current internet age we have pseudo credit scores I call them e scores because
they're electronic they're done on the fly by websites or by companies they decide whether we're good or bad consumers or customers there's no law they're they're not subject to any law that's anti-discriminatory so they can use whatever information they want about us including like our race including who our Facebook friends are etc and we have no access to that data nor can we complain if it's wrong so it's just it's like at the wild west in some sense we're going back to that that banker who's just who's looking us up and down and deciding based on totally subjective information whether we're worthy and this is by the way I should say that these e scores are not being used in exactly the same way as the FICO scores the FICO scores you know there's if you're actually being offered credit like through a credit card then it is subject to those laws I mentioned but this is the way that the e scores are being used if you go to the capital one website
then an e score they will they will perform sort of they will profile you and decide whether you look like a high value customer or low value customer and depending on which kind of customer you look like they will populate their website with different kinds of ads so no it's not a it's not a credit offer but it is the advertising environment that you enter into like so if you if you look like a high value customer you're going to get a like an advertisement for a fancy credit card with probably better deals then if you look like a low value customer and again it's not it's not like fair there's no reason to think that they're accurate they're just doing it because they can and because it's efficient and profitable and and the data if I understand correctly that they're using is not that the the objective and and unarguably relevant data of your past credit performance they're using these things that you describe in a lot of these algorithms that are being used to
decide so many things in our lives they're they're using proxies which are information that is supposed to approximate your credit performance but may or may not be relevant is that is that a very good answer absolutely yeah I mean like again I don't I don't want to say that I'm not a huge supporter of credit scores especially as FICO scores are being used as proxies themselves for whether you're a moral upright citizen and and many employers are allowed to look at credit reports to decide whether to offer you a job and I think that's inappropriate if you think about the feedback loop of people who have bad credit reports because they are out of work and they need a job and it's keeping them out of a job because they have bad credit reports it's like a terrible cycle so I'm not gonna I'm not gonna argue for FICO scores as the best tool ever um but one great thing about FICO scores is that the data they use to build your credit report is relevant to whether you're going to pay your bills it's questions like do you pay your
electricity bill do you to pay your medical bill um and that's I feel like that's kind of a fair set of data to look at when you're thinking about loaning somebody money um by contrast the data that is available to say capital one website when when you browse to them it has nothing to do with whether you've paid your electricity bill it's mostly things like where you on Facebook what does your Facebook profile look like um do you where's your location where are you living right now or where's your um computer situated is it in a poor part of Harlem is it in a ritzy part of the of a upper aside that kind of question so it's a very demographic based and behavioral consumer behavioral based um and so it doesn't amount to much more than the the same thing we were talking about with the banker it just sort of sort of profiling you and and it happens in a heartbeat right it happens in milliseconds yeah so I mean people sort of think oh I went to the website you should go to the website as if it's a thing it's not a thing it
it is rendered when you get there and all these decisions are made based on what they who they think you are as a consumer and one of the criteria as I understand your definition of weapons of math destruction for for something being a wb is not only the effect that it has on people whether they get jobs whether they get credit whether they get a college education whether they manage to climb a ladder out of their particular circumstance to a better one but it's also the fact any you touched on before that the data that's going into these algorithms is secret and proprietary and it's a black box situation right and I think they go hand in hand I think like the fact that this kind of demographic profiling can be allowed to happen is because it's secret I think if we had view into these practices we would say hey that's obviously a bad idea that's obviously profiling it's discriminatory and it's the opposite of mobility as you point out like what are the
chances that you're going to be able to climb out of the your sort of poor situation of birth if every time you look somewhere every time you try something an algorithm deems you as a likely loser and prevents you from getting something I mean that's really what I'm talking about and when I say my subtitle of my book is how big data increases inequality and threatens democracy and when I say increases inequality I mean that there are algorithmic forces at work and they are they are separating the winners from the losers as I did after I left finance and joined data science I was separating winners from losers but I was separating them in the same old way that we used to separate them through class and race and gender and all these algorithms are doing the same thing and so it is a kind of invisible but I believe very potent force of inequality and it is it is squashing what's left of the American dream talk about buckets and tribes because we're not just being separated into two categories we're being separated into dozens and hundreds
right that's right I mean so I as I said I was working as a data scientist after I left finance and I I was doing something I considered relatively benign I was deciding whether people on Expedia.com deserve to see an ad and I was like okay well whatever nobody's going to I know I know nobody deserves to see an ad the idea was if they if they were going to buy then I wasn't going to show them an ad because it would have taken them off the website but if they weren't they didn't seem like a likely buyer then at least we could sort of get the three cents that we've got paid to click on the ad so who who was deemed worthy of this ad and again I was like this is not this is not big potatoes right and then a venture capitalist came to visit our company he was thinking of he was thinking of investing series B round funding and he had us all sit down listen to his vision of the future of the internet and okay venture capitalist he's an architect
right he's he's the guy who decides what gets funded and what doesn't so he has he has influence and his vision was this he said I can't wait for the day when all I see are ads for trips to Aruba and jet skis and I never see another University of Phoenix ad because those aren't for me those aren't for people like me and like the people around me laughed and I was like dude like this is dystopian this is the opposite of the internet as a democratizing force this is actually the goal here the goal is to stratify everyone by class by gender by race like get like I want to be given opportunities let's leave it to other people to be preyed upon and that's that's what for profit colleges do they prey upon people and they specifically target and I did research on this after he said that because I should say like I had never seen a University of Phoenix ad I didn't know what it was I I had to go incognito mode to even get a University of
Phoenix ad because of course I was a highly educated white woman in a Tony part of Manhattan with a great job I wasn't targeted by for profit colleges and yet I found out that the for profit colleges that the University of Phoenix in particular the parent company Apollo group was the number one Google ad buyer that quarter it wasn't a small deal this was like the biggest advertiser on Google and moreover I found out that for profit colleges you know have a very low graduation rates but even if you do graduate a diploma it's not worth more than a high school diploma and it's sad all their students with enormous amounts of debt didn't give them much of an education it was a it was a scheme it was really a way of gaming the federal aid system and calling it education and I realized that you know I was contributing to it I felt complicit yet again I felt like a co-conspirator like I had in finance so I felt like wait I
I think what I'm doing is benign but of course I'm good at my job and nobody stays at any internet company for more than a couple years so I I developed a technology and then I share it with the people I work with and then they move on to their next job and they share that with people they're working with and at the end of the day I'm contributing to a system where other people are suffering but I am never suffering that I am I'm only benefiting from and that's kind of how I started seeing the world of technologists and data scientists in particular that we're building a universe in which we're the winners sounds like the old system well maybe with it's I don't think it's a different from the old system but it the the sort of at least temporary differences that we're calling it objective we're calling it innovative and and we're we're bragging about how great we are at intelligence machine artificial intelligence and how everybody should be on board because like machines can beat go players you know I think it has a pretty good marketing
department yeah technology company executives have been cover boys of business magazines now going on 25 years as heroes so these these categories that we're put in for let's say the marketing purposes of getting either a group of vacation ads or or University of Phoenix ads they're based on our behavior as the internet perceives it as as devices that crawl the internet perceive it is that where the information is that where the data is coming from our our performance our our activity on the internet I'll draw you a landscape of the of the data industry the data marketplace there are three big companies in the world in this world there's amazon amazon doesn't sell your information it collects your information as you buy stuff there's Facebook
Facebook doesn't sell your information directly although it sells categories of people to advertisers and that's how it makes money so it doesn't sell doesn't say hey caffeine is a woman with PhD white living in New York it doesn't do that but if it if you want to advertise to white women in New York at who's four or 45 years old then you'll find me right they also I think buy information from data warehousing companies which we're going to talk about in a second and then there's Google who doesn't sell your information either but does indirectly sell your information to advertisers through categories like Facebook and I don't think Google buys data elsewhere and then outside those three big companies who are like islands that collect data they don't sell data they collect data and they use data but they don't sell it then there's just an enormous industry of second hand data markets which is to say if you go to sort of any site outside those three those three companies there are things called pixels where where third-party data gathering companies will bombard you with cookies and they'll track you and they'll see oh I saw you
at footlocker.com and now I'm seeing you at zazzle.com whatever it is and then they collect all this information about you there's hundreds and hundreds of those companies they do that and they sell that information to big data warehousing companies like Axiom and Axiom is one is I think the biggest data warehousing company that collects all those information as well as scraping public records and then it creates profiles for every consumer in the country Axiom has a profile in everybody and then Axiom then it's a middleman and it turns around and sells those profiles to companies that are interested like insurance companies probably by that probably large employers like Walmart I don't know exactly who the customers of Axiom are because it's a secret so I don't want to say something incorrect but that's they are the middleman and they are they're a very profitable company that that buys data about you and sells profiles to other people and this may not be in your wheelhouse but does do not track does tracking well ad blocking does all these devices that have
grown up to supposedly grant the internet users a centilla of privacy they keep you out of that marketplace or does that just thin your your data flow to the data merchandisers so ad blockers doesn't thin anything ad blockers just doesn't let you see it at the end of the day at the advertising but it doesn't prevent a future potential employer by to buy information about you so ad blockers just is a way of kind of ignoring something but it doesn't stop tracking as far as I know it all I might be wrong about that there's the do not track you can you can set your browser to do not track it it is not it's not honored nobody honors that as far as I know it's just ignored so that's your you're asking nicely and they're ignoring you you're tracked but I'll say I'll say something which is that I don't I don't really care about that for for myself and I'm going to go
back to what I was saying about the VC and what he made me realize is that this system isn't going to hurt me I mean I'm not saying it will never hurt me at all but I'm saying it I'm not the victim here the victims are the people who have their demographics working against them and multiple ways and they're the ones that are in every algorithmic system deemed the losers so going back to for profit colleges for a second they're not just looking for poor people they're looking for poor people that are also unaware of how college works so they don't for specifically for people who don't know the difference between private colleges and for-profit colleges they think public colleges sounds not as good as private or for-profit and there are people like this and they're they're often like parents of immigrants they're often you know people whose parents didn't go to college in general right so they're they're ignorant in a certain sense and they're good but they know the one thing they do know is that if they want to be a real citizen of
the middle class they have to go to college so they are vulnerable to the pitch and so it's it's you're saying about tribes it's not just that they're poor they have to be poor by the way because otherwise they won't be eligible for federal aid and this as I said it's a federal aid gaming system nobody who's not poor would ever be approached by for-profit colleges they only interested in that but it's not just that you have to intersect two things you have to intersect being poor and being ignorant so that's that's where you really get the predatory behavior and you it's not just for-profit colleges you also have payday lenders doing exactly the same kind of thing vulnerable looking for vulnerable people who have no other options low information voters I think the phrase is in some circles yeah that's sort of equivalent in the in the world of politics yeah but there's something else that goes into the mix for for-profit colleges isn't they're a desire to get ahead improve yourself I mean that's that's got to be measure is that measured in some way is that part of the the tribe that you're you're put in by the by the data
minors or does that show up anyone certainly certainly yes absolutely I mean in in in fact like the real way this works is you know the way Google ads are sold is by auction and by keywords so definitely the four-profit colleges are paying big bucks for keywords such as college go to college where can I go to college you know phrases like that but they're particularly interested in a certain demographic yeah so in a sense you're being punished for being ambitious but in the wrong demographic exactly it's a fascinating book and you have some suggestions at the end for ways to deal with this so that the picture is not all bleak have you had any feedback or brush blowback from the industry after this book was published you know I haven't I was expecting more than I got I I've gotten a lot of sheepish data scientists saying wow I'd never really thought about it I've got a lot of silence I'm starting to feel a little pushback from the tech
giants indirectly I think there's just an enormous amount of interest right now in antitrust law and whether it can try to push back against the power of Google and Facebook and I have strong opinions about that one of the things I call for near the end of the book with respect to political ads is like that fact that we we have no idea what kind of messages are being sent to people on Facebook and we even had voter suppression ads they were the only reason we know about that is because the Trump administration the Trump campaign actually bragged that they were sending out voter suppression ads to African-Americans on Facebook to keep them from voting but we haven't seen what they say maybe maybe their false information we have no idea there's it's complete it's a completely opaque so I was calling for you know show us show us the ads show us all of them let me as a journalist look to see if you're sending different messages to different people if
you're sending false information to people what kind of manipulation is this and we know it's propaganda that's kind of understood well advertising is propaganda yeah and political advertising yeah but the I think it just it enters a even more dangerous territory when you can when you can tailor your message to exactly the person who is going to see it and no one else is going to see it and that's why I worry about that so just this week I realize I heard that Facebook has agreed to do something along these lines and that's really good news you know I'm reminded as you as you talk about that of another book I read recently which is Tim Wu's book about the attention merchants and yes basically what you're describing is what advertisers have been trying to do for a hundred years now which is a to attract our attention and be to talk to only likely prospects exactly and technology has been you know sort of a golden window into that promised land for them not for us for them not for us I would I would argue that we should just make it illegal to
tailor political advertisement I mean I feel like we've already got enough evidence that it's a bad idea like we should just not let them decide exactly who this is going to be seen by we should say you can show the ad to everyone or no one or a random selection of people but you can't decide who to see who should see this and and yet they think that obviously of course and the advertising trades tell me that television networks are now trying to ape the internet in being able to more closely target viewers for the purposes of advertising targeting so that that's a battle that may be on a fought on a larger battlefield than just the the internet itself we're we're running out of time Kathy and I'm so glad you you shared your information and your insights with us this hour it's a fascinating book weapons of maths destruction by Dr. Kathy O'Neill. Thanks for having me my pleasure thank you
you well ladies and gentlemen that's going to conclude this week's edition of the show program it turns next week same time same audio device of choice a tip of the show shout out to the San Diego Pittsburgh Chicago and exile and Hawaii desks for pretty much being on standby this week
thanks as always to Pam Hallstead in Santa Monica to Jenny Lawson somewhere on the east coast in North America to Noriko Okabe at Argo Studios in New York City and Jeffrey Talbot at audio works in New Orleans for help with today's broadcast the email address for this program your chance to get cars I talk t-shirts for the holidays don't you think and playlist of the music heard here on all available at harryshear.com and I persist on Twitter at the harryshear you
The show comes to you from Century of Progress Productions and originates through the facilities of W.W.N. on New Orleans flagship station of the Change is easy radio network, so long from London.
- Series
- Le Show
- Episode
- 2017-11-27
- Producing Organization
- Century of Progress Productions
- Contributing Organization
- Century of Progress Productions (Santa Monica, California)
- AAPB ID
- cpb-aacip-e1882dba120
If you have more information about this item than what is given here, or if you have concerns about this record, we want to know! Contact us, indicating the AAPB ID (cpb-aacip-e1882dba120).
- Description
- Episode Description
- No description available.
- Broadcast Date
- 2017-11-27
- Asset type
- Episode
- Media type
- Sound
- Duration
- 00:59:03.196
- Credits
-
-
Host: Shearer, Harry
Producing Organization: Century of Progress Productions
Writer: Shearer, Harry
- AAPB Contributor Holdings
-
Century of Progress Productions
Identifier: cpb-aacip-3cce81af2e6 (Filename)
Format: Zip drive
If you have a copy of this asset and would like us to add it to our catalog, please contact us.
- Citations
- Chicago: “Le Show; 2017-11-27,” 2017-11-27, Century of Progress Productions, American Archive of Public Broadcasting (GBH and the Library of Congress), Boston, MA and Washington, DC, accessed May 14, 2024, http://americanarchive.org/catalog/cpb-aacip-e1882dba120.
- MLA: “Le Show; 2017-11-27.” 2017-11-27. Century of Progress Productions, American Archive of Public Broadcasting (GBH and the Library of Congress), Boston, MA and Washington, DC. Web. May 14, 2024. <http://americanarchive.org/catalog/cpb-aacip-e1882dba120>.
- APA: Le Show; 2017-11-27. Boston, MA: Century of Progress Productions, American Archive of Public Broadcasting (GBH and the Library of Congress), Boston, MA and Washington, DC. Retrieved from http://americanarchive.org/catalog/cpb-aacip-e1882dba120