Le Show; 2018-03-04
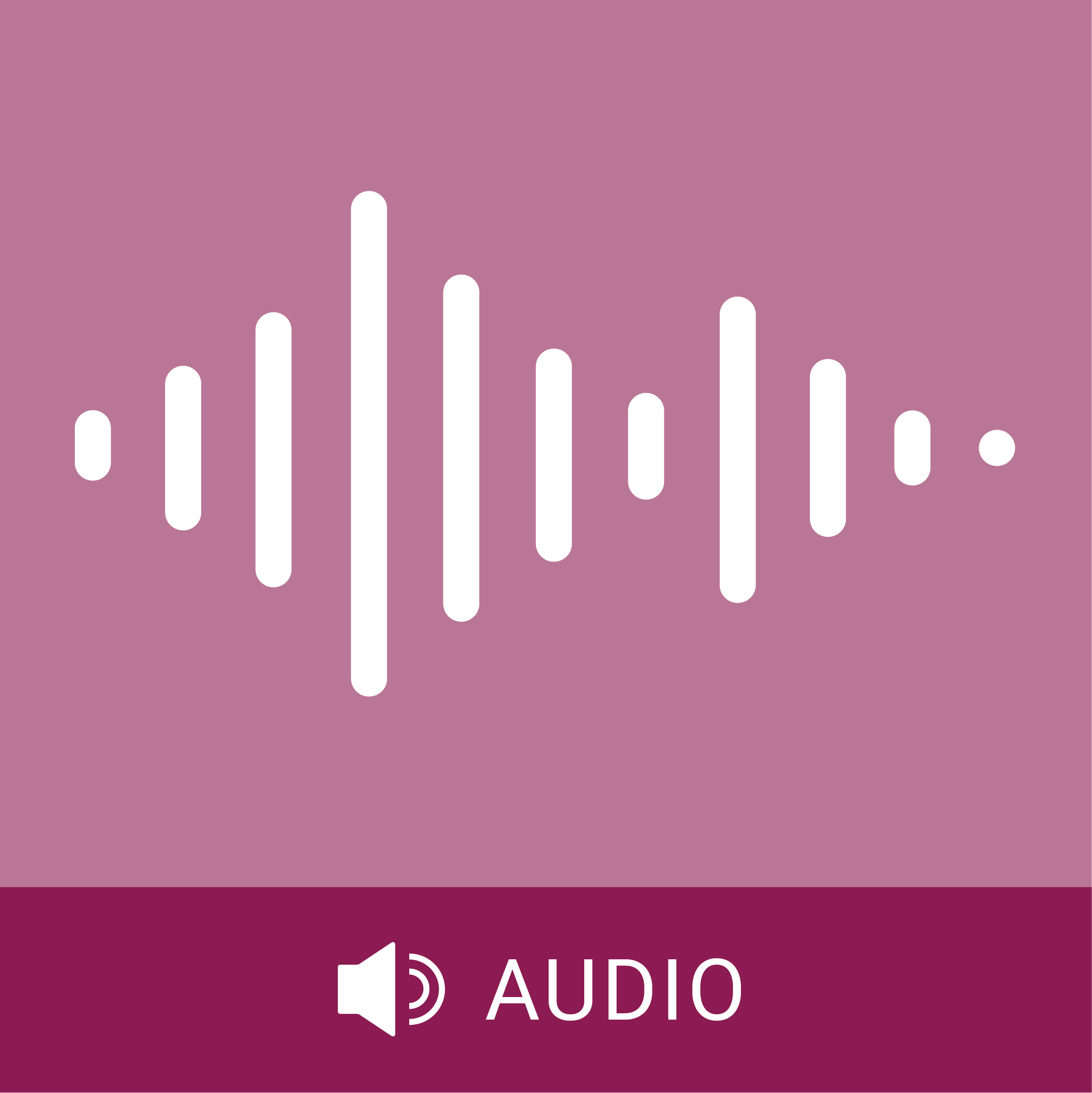
- Transcript
From deep inside your audio device of choice. This is Lesho and this program is building in a way and moving onward from a conversation on this broadcast last November I think with Cassio Neal on the subject of algorithms. And today we go broader and deeper into the world of artificial intelligence and to talk about it as the most artificially intelligent person I know, his name is Gary Marcus. He's a scientist, best-selling author, entrepreneur, CEO and founder of the machine learning start-up Geometric Intelligence, recently acquired by Uber. He's a professor of psychology and neural science at NYU and his books include The Algebraic Mind and Cluj, the haphazard evolution of the human mind. Cluj, Cluj, people vary but I would definitely say Cluj, then you would and that's Gary. Gary, welcome to the program. Thank you very much for having me.
Now let's start I guess for people who have just heard this term incessantly hyped and critiqued in the media. What is in this current state of the thing artificial intelligence? I think artificial intelligence is a very vague term. I'm not going to try to protest otherwise but the notion is you want to take machines and make them do intelligent things and it's a research question and it's a applied technology. You see it in things like Siri and someday you might see it in things that are clever as the Star Trek computer. Right now I think there's more hype than reality but finally for the first time there is some reality. So we have practical everyday products like Siri and Alexa that have at least a little bit of artificial intelligence. They use that artificial intelligence for example to understand speech, they don't necessarily understand the words that you mean but at least they can kind of transcribe them into written speech and then they can operate over that speech and understand a lot of basic questions.
That's one form of artificial intelligence. You also have artificial intelligence for example in robots to help them move, help them look at things, help them make decisions. When you say Siri and Alexa, the two fates can understand, when they parsed your spoken or written speech, they're basically responding with scripts, right? They're not responding spontaneously based on an actual understanding of what you've said. Is that a correct understanding of what they're doing? It's a mix nowadays. They certainly don't have a full understanding like human being would have. They're going to be some things that are very scripted like their wisecracks, like if this is still true but you ask Siri, what's the movie Blade Runner about and they'll say it's a film about personal assistance, digital assistance and some human wrote that wisecrack. It's not like the machine watched Blade Runner understood what was going on, analyzed it and then came back with a reply appropriate to you. On something else, maybe a year or two from now one of these personal assistance might
be able to say in response to how much does it cost to fly from New York to Boston, they might be able to say there are three flights available and they cost this much. I don't think any systems quite good enough to do that reliably yet but when systems do things like that, they're trying to parse your sentence into some kind of frame like somebody made a request to travel from ex location to why location they're interested in the cost. There's little bits of that that are templates I think is a good word for it and so Siri is going to be an amalgam of templates and kind of almost like canned replies. And then they have a human voice generator that makes it sound like there's a person. That's right. That's what we call text-to-speech and people have been working on that for 50 years at now. It's pretty good. I think it's fair to say a fall on the side of the line of a skeptic about where AI is where certain AI technology is today and whether it's got a future full of new skills
and capacities, what is the basis for your skeptical attitude? Why are you such a sc... Why are you such a nascar? Why am I such a crank? Well, first I should say, I'm not that skeptical about the distant future. I think that 100 years from now we will have AI systems that are pretty clever. 100 years from now we'll all be dead, mate. Well you and me, but hopefully not all of mankind are humanity, so we could make mistakes. If such mistakes are not made, then I think AI is just going to get better and better. But I think that a lot of people like to extrapolate pretty wildly and they say, look, I see this one little problem is solved, and so I think all problems must be not very far away. I call that the ladder to the moon fallacy, right? I've built it, I didn't make it up, but I've built a ladder and I think next week I'm going to get to the moon.
Well, it turns out that the ladder is not the right technology to get to the moon. We have some very good technologies right now for doing certain problems like speech recognition, so identifying those words, and not very good technology for other things like understanding your sentences. So you can't have a conversation, a real conversation with any of these things, Siri, Cortana, Alexa, et cetera. They didn't know where near to really genuinely understanding your sentences. And there hasn't even been that much progress on that. So there was a program called Eliza in 1965, and people would talk to it and it pretended to be a psychotherapist. They would talk to it by teletype, which is basically like text messaging. And some people were fooled. They didn't understand. There could be a computer on the other end, and they would explain about their problems with their boyfriend or their mother-in-law or whatever. And the machine would use very dumb rules to reply, like if I'd heard the word mother-in-law would say, well, tell me more about your family. And people were, you know, gradualist and gullible, and they thought they were talking to the machine, but they weren't really. So they could have this conversation, and the machine was programmed to keep the conversation
going. But it's not like at the end of the conversation, the machine could kind of put together all of what you said and say, well, this is what your problem is really about. You know, it's like a perfect Freudian analyst. Oh, well, I would say that the perfect Freudian analyst is actually trying to understand you. And this system is like, you know, trying to give you, you know, witty repartee at best. And so anyway, Eliza was built in 1965. Siri is not that different. You know, Siri is still like, there's some pre-planned witty repartee, it understands a few keywords and so forth, but the genuine understanding of like what human conversations are about. So there are concepts of like family relationships and dynamics between individuals. You know, people might be worried about money, how economics work, any of that kind of stuff. We don't have machines that understand those kind of basic everyday concepts. And so everything they do is like a bit of fakery right now. And there's been a lot of progress on the fakery, but there's been almost no progress on genuine comprehension.
And so that comes out in the differences between what machines can and can't do. So here's something my five year old is learning to do. No, no computer can really do that at this point. So he can read a basic story and tell you like who did what to whom and why. And you know, no computer system can get matched what a five year old can do. Or you know, look at the, I have a three and a half year old and you can look at what she can do in terms of her body and like she's, you know, starting to learn to ski. It's very difficult to get robots to do anything like that without enormous amounts of practice, there is humans, you know, in a day or two of practice can start to pick up new skills. You mentioned the word fakery and I want to take a little tangent here and bring up something that's in the news, which is something called deep fake. And there's a piece on the law fair blog currently about the potential of deep fakes. I'm, I'm presuming you're familiar with the, the term and what it, what it means. Frightened by it.
You are, you are frightened by it. Yeah, I mean, I, I think, I mean, basically the idea is, is to fake human voice, fake human video and we're already to the point where you can fake voice to some extent and we're just going to get better and better at that. So that's actually in a sweet spot of what machines can do. And so we're going to more and more see, you know, somebody putting words into Donald Trump's mouth or put into my mouth or what have you. And you're going to look at the video and it's going to look real, but it's not going to be real. And we have no great means to combat those things and there's going to be cat and mouse games. So some clever person will figure out that all the fakes, you know, the seventh and eights pixel do this funny thing. That's not a real example. And the people who make the fakes will just change the seventh and eight pixel then they'll be back to the drawing board. So it's going to be much like we see in cybersecurity in general. People figure out how to make some kind of computer virus and we figure out a short term patch and then they figure out a different way to do it. There's no magical solution to this.
So the doors for the deep fakery of the world have opened and they will not be closed again. Well, you know, I read these alarmed stories about how deep fakery will erode the last vestiges of trust in information and think we've been living in a Photoshop world for 20 years now. And it's relatively easy for even a ham-handed person like me to use Photoshop to make, let's say, a figure in a photograph smile when that person wasn't smiling. And yet we still believe in the power of photography to tell us something useful about the world. I mean, we're actually too trusting of the power of photography because Photoshop is a very powerful tool. I mean, to do it well and really fool an expert is hard, but it's not impossible. You know, often there are telltales or, I mean, you know, magazine covers have people with three hands on them sometimes. The fakery is not always perfect and there's no algorithm to do it perfectly well.
But the algorithms for doing that have actually gotten a lot better and you know, there's what do they call it, deep porn now or something like that where you can, you know, take one person's body and another person's face and you can make a hybrid of it. If it's not fully convincing yet, I can't say I've actually looked at the materials, but you know, if it's not convincing this week, it will be in a year or two years. It's not so far beyond the understanding of the kind of technology that we can build. Let me get back to the main point here. The brain, the artificial intelligence is trying to replicate some of the functions of the human brain, right? Yeah, I mean, I thought you were going to say, replicate the human brain and I wouldn't say that, but what you said is more careful. So a lot of times I get in conversations where people are like, well, why do you want to replicate humans? And we don't want to do that. Those of us working in AI, you know, I already built two little humans that kids I mentioned and that's better.
It was fun. And it was fun, but it doesn't mean I need to, you know, build a robot to do exactly the same things. But there are things I would like robots to do that I might not want my kids to do and I would like, you know, the robots to be clever enough and that there can be a range of reasons for that. They can be dangerous, for example. I don't want my kids in Fukushima trying to fix the nuclear reactor. I don't want your kids there either. If I get a robot that can navigate a human environment where there's bright, high radiation and we don't want people to be there, well, that's, you know, that's a win for society. Although the robots in Japan have been forced out of the, because they can't take the radiation level either. Well, I mean, you can get to situations like that. I actually have a friend who builds robots to land on Venus and the, you know, you can't stay there for very long. I don't remember the exact details, but it's something like he's trying to get them to land on Venus for 12 minutes before they melt instead of two because you get a bunch of scientific data you couldn't otherwise get, you know, 12 minutes is a win in that project.
So, you know, if you get one robot the last 12 minutes in Fukushima and it saves, you know, five lives, then that's great. So there are lots of things we would like robots to do, but they're not versatile enough, right? And just to make something explicit, robots are driven by artificial intelligence. There's a hardware component, the software component is the artificial intelligence. So there are a lot of reasons why we would like to have AI that's flexible that can, you know, what's cool about a person that you could send into a place like Fukushima, you don't want them to have to go there is they can, you know, deal with all these kinds of things like climbing ladders, opening doors and stuff like a human and a junior environment. They can do, you give them instructions and say, let's put this thing together in this way. Like, I don't know if you saw the movie Apollo 13, but there's that amazing scene based on reality where they had to figure out how to make substitute air filters or connect the round ones to the square ones and they use like socks and duct tape and so forth. You don't want to have to train for a million trials on how to do that. You're not going to get the data, the problem with current AI is it's extremely data hungry. So it doesn't work unless it gets millions of trials.
You want something with a versatility of a person who can get those instructions verbally from Houston and be like, okay, so I'm taking the duct tape on wrapping around, what do I do now? How many times do I go around? Three times. Okay, great. And we don't have robots that can take human instruction or work with human materials in a flexible way, but when we can, it's going to be great for elder care and societies where the demographics are such that we don't have enough people to take care of them, they're going to be great in this kind of Fukushima situation. Then there are other things like we would like AI to be able to read so it can compile the scientific literature. Like I'm a scientist, there's no way I can keep up with all of the scientific literature anymore. It's just impossible. If I had a bot that could read everything in five different areas and distill that for me every day, that'd be amazing. But you're saying we need artificial intelligence to recreate the reader's digest. That's one of the reasons, yeah, and not just the reader's digest, but I'm talking about synthesis. I like readers' digest is about summary, but what a scientist does is they have to distill many papers and put them together and say, how does this all fit together?
And that's complicated because, for example, the results aren't even all consistently that there's noise in different experiments and so forth. So anyway, going back to an earlier question, I don't want to copy human beings, but I do want to learn something from their brains because human beings do some pretty cool things, like they understand explicit language, and they're very flexible. They can learn new problems in like a minute. I can make up some game, like let's play cards, and every time you see the third card, I want you to touch your head to your table, or bounce your head against the table, you can do that. There's something about you that's very flexible, where's the machines that we're using now need like millions of training examples, often underpaid humans have to label a bunch of things, and then they have no flexibility, they work on that problem, so deep mind is famous for their system that plays go really well, but that's all it does, and they figured out a way to get it to play chess too, great, but that doesn't mean that it's going to be all to play risk or diplomacy, let alone that it's going to be all to help you with medicine. So we train like Harvard undergraduates so that they can do anything, but machines
that we kind of like, we're happy if we give them six billion training examples, and they do one thing well, and so there's something to learn from people. Recognizing dog from having been shown two million photographs of dogs is what you're talking about. Yeah, machines are great at that now, and you can train a machine to recognize different dog breeds, probably better than at least anyone who's not a dog lover, and I mean that's useful, and there are a bunch of applications over that, the same technology drives speech recognition, so you can learn to recognize syllables, you know, Alexa's ability to recognize speech from across a room with echoes and stuff like that is pretty spectacular, and that comes from using a lot of training data in different kinds of environments, they were very clever about how they did it. So that's something where you can get a lot of data, and it's very useful, so it's not that we can't do anything now with AI, but there is this kind of flexibility where we encounter new problems, human beings are better at that, and like I would like to know why they're
better at that, so I can make the AI better. This is basically the problem of extrapolation. Well extrapolation is indeed one of the fundamental problems I think machines aren't good at, so you can think if I have a bunch of data, and I'm trying to guess what happens to the next piece of data, and it's in between the examples I've seen before, though let's call interpolation, and current AI systems are pretty good at that, but human beings can do something else called extrapolation, where you go kind of outside of the boundaries of the data that you've seen before, so I give you a sequence, I'll give you a very easy one, two, four, six, eight, and even if you haven't heard the continuation, you can guess, ah well these are even numbers proceeding by two, and I'll go with 10 as the next one, even though that's not in the range of the numbers that you've seen before. So that was the right answer. That was the right answer. Actually, there's an interesting philosophical puzzle there, so it's called the problem of under-determination. So actually, there's no right answer, but there's a, how do I put it, there are some answers that at least to human mind would make more sense, and if you, there's no right answer
in the problem that I pose, other than Gary feels that tends to be the right answer, but there can be real world problems where you do want to extrapolate, so let me give you an example of that, that's less artificial, which is you can see a whole range of examples of people driving in different situations, you record the data, and you could try to just mimic what humans have done when they're driving. And maybe that gives you a lot of different conditions on the highway. And now I take you off the highway, well that's extrapolation, so that you have to do something outside of what you've seen before. And some of the problems we've seen with driverless cars are really extrapolation problems, so you know a Tesla drove into a stopped fire truck the other day at 65 miles an hour. And I mean, no human would ever do that, and human is extrapolating maybe beyond the range of experience. Most of us probably have not seen stop fire trucks on the highway, you know, I hope most of us don't have to have that experience. But we know something about physics and you know large objects that aren't moving and so forth, and we don't have to use such aggressive technique called filtering.
And so we see the fire truck stopped and we're like, hey, that's a problem, I should deal with that, even though it's not directly in a realm of our experience. So there's an extrapolation where you could say logically relative to all the data I've seen before a fire truck could be anything, but if you have any sense, you'd say a fire truck stopped on the side of the road, I should get out of the way. I had this very experience not with a fire truck, but with a fallen tree the other night. And my first perception was gee, the painted delineation of a lane has stopped. That was the very first perception I had was, why did they stop painting the lane mark? Oh no, there's a thing, a tree hit the brake. And what you do there is reason about it. You're like, what are the thing, you know, what could have caused that? Why is that there? You ask a lot of why questions implicitly. And the machines don't do that. It's more like, here's a bunch of statistical correlations. What have I done in this circumstance before? And always asking, what have I done here before? It's not always the right question because you encounter, as you said, something you haven't
seen before. That's when you need to use the why questions that my three-year-olds are in, my five-year-old is asking all the time. That's what's missing is the ability to reflect and ask, what are the causal principles? Why is it this way? You know, there was a paper written by Stephen Levy in back channel, maybe, a couple years ago, or an essay, where he went and visited Google. And Google has like a little, not a town, but like a little area where they test driverless cars. And he went around and he got, you know, introduced the people working there and did a nice journalist thick piece, you know, explaining how the guys were trying to build a driverless car. I think this is before they spun out into Weimo. And the kind of hooker of the piece or whatever, the bright moment, the climax was they learned to not stop prematurely every time they saw the leaves on the ground. It was like the giant accomplishment of this research team and the months or whatever that Levy was visiting them. And like, there's something wrong with that. It shouldn't take you months, enormous amounts of training examples just to recognize leaves.
And then if you've done all that and then there's a pogostick on the ground, are you able to deal with that? Well, then you've done all that work and then it's winter and the leaves aren't on the ground anymore. So it's kind of wasted of time. I have, you bring up driverless cars, which is a context in which we've talked because you've been involved in this work for some time. Have you seen the story about the people at Princeton who devised a way to spoof? There's the stop sign, speed limit, one. It's actually a KFC sign to fool whatever was running the car into thinking it was a stop sign. Yeah, there's a whole bunch of things like that now. So there's a whole literature on spoofing and what people who work on this field called deep learning will say is, well, that's just like an optical illusion and people are subject to optical illusions, too, and what's the big deal here?
But what I think is going on is actually much deeper than that. So you have all of these cases where it's very easy to fool these systems. Another one, people can look up later or you can put in the show notes if you have those, is called adversarial patches. And these are like, you show a deep learning system, a picture of a banana and then, or a real banana and it says banana. Now you put a little sticker, tiny sticker that has a toaster on it. And a human will say, well, that's a banana with a sticker with a toaster on it. And these systems will say toaster if you make it, you know, if you make the toaster shinier than the banana or something like that. So they're looking for like, what's the most salient thing in the picture? And you're looking for like, what's going on in the picture? Oh, I see somebody put that on the why, but they put a sticker on top of the banana, isn't that unusual? And so the KFC thing that you're talking about is probably similar. I don't know the exact stimulus, but I can imagine, for example, you take a KFC sign, you put a couple stickers in the right place or the wrong place and now your driverless car thinks it's a stop sign or you could make it think it's a speed limit sign or a road
or a road. I'm looking at it. And it's a very subtle manipulation of the of the kernel logo, putting a bit of bit more red in his face, seems to have fooled the machine in this particular. Yeah. I mean, that's scary, right? And that's again about kind of interpolation and extrapolation. So maybe you have never actually seen a KFC sign that's quite that red. And you're not going to be thrown by that because you still have, you know, reference point about what a KFC sign is it because I still know what the kernel looks like. Exactly. And these machines, it's like, they're just, they have a bunch of memorized pixels. They don't, they don't know from, from kernel sanders. And they should, they should thank their lucky bits. You were in a debate recently at NYU with a colleague and you, the two of you seem to fall either by choice or by nature on different sides of the question of learning in the human brain.
And therefore in the machine brain, dependent on a lot of innate stuff that sort of comes with the factory supplied, so to speak, or is it all a bunch of experiential learning? And you fell on the innate stuff side if I, if I don't mischaracterize you. That's right. I mean, there were actually two debates going on there. There was the one we're supposed to have in the one that we had anyway. So the one we had anyway was, do humans have a lot of factory installed software? And I think the answer is absolutely yes. Another one of my books, he didn't mention at the beginning is called The Birth of the Mind. And that's all about how a small number of genes, you know, we only have like 20,000 of them, can manage to build the complexity of the first draft of the human brain. And the human brain has 80 billion neurons. And I talk about, among other things, the way that the genome is kind of compression scheme, in the same way that like you can have, you know, a hundred K pixels that describe a picture that has many more, if you look for all the redundant patterns and so forth. The genome is brilliant at taking redundant patterns and making a lot of structure. It does the same thing in building your heart as it does in building your brain.
You're innate, I'm sorry, the structure of your heart is innate, it's built in. You can change it by doing a lot of aerobic exercise, you make your heart bigger. But the basic design is built in, and I think the same is true for your brain. Lecune does not, for reasons I didn't think he gave any convincing reason, why not? But he didn't believe that it was. And then the debate we were supposed to have is take that same question and ask for artificial intelligence. How much should artificial intelligence have built in if we wanted to be effective? So Lecune is one of the big players in deep learning. He's one of the three founders of the field. And until recently he was directing Facebook AI labs, now he's the chief scientist at Facebook. Lecune's position is you don't need anything built in. You just need powerful learning algorithms and you'll get where you need to go. My position is there's really good reason why biology has built in a lot of stuff, I think. Of course, we can talk about natural selection, it's not with purpose, but the things that have been selected for have a lot of innate structure.
And I think that's largely what the genome is for. And I think people in AI are kind of foolish to overlook that lesson. And then there's some more subtle arguments. I actually wrote an archive article, AARXIV, about AlphaGo, which is DeepMind's famous co-playing systems and innateness in January was a little bit after the debate. And I talked about how in systems that work, people actually build in a lot of innate structure. They often kind of hide it and pretend that it's not really there. It's kind of like off stage, you know, the famous line, Payno attention to the man behind the curtain. So there's a lot of stuff behind the curtain number one. And number two, they get these systems to work with all the stuff behind the curtain for one little problem. They don't get them to work generally. And who's to say that we won't need a bunch of different stuff built in when we go to the next problem? And so I think what AI should be doing with researchers in AI should be doing in part, not the only thing they should be doing, is saying what are the prior things that if you built those into your system would allow you to learn more efficiently. So I just posted on Twitter today a video, a link to a story about, or a research report
about baby octopus, a Dumbo octopus. And within like 10 minutes of when it's born, it can navigate around the world and do all of these interesting things. So the octopus has a bunch of things built in and helping it to understand space and time and maybe predators and prey, stuff like that. Natural selection is, you know, weeded out the octopus that can navigate the world. Well, maybe for example, when we build robots, do we want each one of them to learn from scratch, everything about how to navigate, or do we want to build in a bunch of navigational tools? In certain parts of applied AI, people take this for granted. So nobody has your GPS system learned from scratch how to navigate. But researchers in AI think it's like too much heavy baggage to build into, you know, knowledge into the machines. It goes back to the old debates that like Plato had about whether there's any innate knowledge and John Locke argued that there's not and so forth.
Practically speaking, biology does in fact have a lot of an ateness. And AI systems that have lacked it have not been that sophisticated. And I think they're excluded. Almost sociologically, there's like a bias in the field. They think it's like cheating to build stuff in. But nature didn't think it's cheating and, you know, creatures like us that have more stuff built in are able to solve more complicated problems than creatures that have less stuff built in. It's interesting to hear you guys debate that and to hear you talk about it now. And almost in the closed box of intelligence, and I say closed box because I think of one always thinks of oneself first. I think of myself having discovered at some early age, oh, they tell me I have this thing called perfect pitch. Well I didn't sit down and have hundreds of hours of training and recognizing what a C was in relation to a G. I knew the first time I heard a note and they gave me and I learned that the names of the notes, oh, that's a G without looking at the piano.
Now that's not something that I was taught. That's something that came in my factory equipment somehow. And talent, talents generally, we find it easy to recognize that that's part of the package we're born with, that some of us are better at freehand drawing than others and that's not because they spend hundreds more hours practicing it, right? Yeah. Another of my books was Guitar Zero, which was about me trying to learn guitar at the age of 40 despite having no talent. And I made some progress, but I did not use that as an argument to say that talent doesn't exist. And in fact, I talked in that book about Malcolm Gladwell's kind of bastardization of Anders Erickson's 10,000 hours. So Erickson actually is very careful to emphasize that it matters what kind of practice you get, what he calls deliberate practice is better than just sort of arbitrary practice. And Gladwell just completely left that out of his book. But beyond that, it was an accurate.
It kind of sold the story that's like anybody can do anything, and it's not true. I mean, talent does exist, and you've got a band like Anvil that's played for a lot more than 10,000 hours, and they're never going to be the Beatles, and it didn't actually take the Beatles 10,000 hours to get as good as they were. So complex skills are always a mixture of experience and some kind of innate talent. In the case of perfect pitch, there is pretty good reason to believe that you have to be born with it, but you also have to calibrate it over time, and there's different versions of it, so some people can do it for any instrument. Some people can only do it in an instrument that they're familiar with. So they're interesting gradations there, and genetics undoubtedly play a role in it. It's an interesting fact that it's perfect pitch is much more prevalent in China than it is in the United States, and some of that's cultural and some of it's genetic. And most kind of complex things are a mixture of a genetic base and experience that expands
on them, and AI is going to be a mix too, right? I mean, there's going to be some stuff that's built in. That's my question. That's my question. Why do they reject by restricting the discussion to intelligence and not having this wider context of skills and performance? Very peculiar. I mean, this is why I actually mentioned the heart. Like nobody thinks that you learn the basic structure of your heart, right? I mean, that would be... Beep now. Beep now. Yeah. You're liver. You know, I mean... How about your liver? That's a very talented organ. You know, the immune system does actually learn something, but the basic structure of the immune system is specified in the genes. And nobody argues that except when they come to the brain, and then it's dualism, like this idea that we have a spirit that's separate from the physical body that's still confessed even, even, I think, very clever people, but there's no actual basis for it. Let me ask you one more slightly philosophical kind of question.
I don't think it's arguable that humans have probably culturally, but maybe innately a preference for explanations of phenomena as opposed to just accepting, hey, we live in a random world to get used to it. Can I give you a word of the day, which you love if you don't know it? It's apophenia, APOP, H-E-N-I-A. I don't know it. Which, as I recall, is kind of like wanting to look for patterns even sometimes when they're not there. Yeah, it's great. Well, I mean, we live in a universe that has both laws and randomness, but we, you know, whether religion or conspiracy theories or science, we want to explain it all, or we feel better if we can explain it all with more or less reliable explanations. Now if we get into a random situation like a drunk driver suddenly appears on the wrong side of an intersection, we also have this built-in equipment, and this is a bit contradictory to the experience I had with the tree that we talked about earlier, but we have instincts,
fighter flight, fighter flight, and shock that keep us from too much rationalizing during the event, that enable us to react more instantaneously than we would if we were just going, hey, that's a drunk driver. I wonder what he drank. You know, we don't go through all that rationalizing at that moment. Now let's take an AI, an autonomous vehicle. Is it equipped at this point in time? Will it be equipped to accept that as a random, uh-oh, as opposed to, gee, now, when have I seen this before, or what would this possibly be, and how much would he have drunk? And obviously in the world of endless data, it could have consumed data about the average amount of alcohol that is drunk in a particular city at a particular time of day, but that's not going to be happening, right?
Yeah, I'll give you another example in a second. I wrote a New Yorker article in 2012 called Moral Machines, and I talked about what would happen if you're in a driverless car, a school bus goes spiraling out of control, and the driverless car has to decide whether to save you at the expense of this school bus full of children or to save yourself, and some people have actually followed that up with studies and interviewed human beings, and Barack Obama wrote about it, and sort of spread pretty far. And it's a very interesting thought experiment. The eventually will, like, play an important role, maybe, in driverless cars right now. It's kind of like the icing on the cake. Like, right now- Right now- It's now- It's now- It's now- It's now- It's now- It's now- It's now- It's now- It's now- It's now- It's now- It's now- It's now- It's now- It's now- Right. You know, is that red thing a truck or not, and you know, the systems are dicey at that.
So, like, it's really interesting stuff, and it's not inconceivable that AI systems might ever be able to do that level of reasoning. But right now, people are, like, racing to get the first, you know, level five driverless car out, and they're, like, cutting corners to show that they're better than the other, and so forth. Nobody is really building systems with that level of sophistication. They're, like, trying to build something that works on sunny days and trying not to worry too much about snow, although I actually just saw an interesting video of Yandex driving their cars in the snow. But, like, the stuff you're talking about is, like, far beyond the level of sophistication that is currently possible. Was it- I believe in conversation or in correspondence on that very- about that very article after I had caught up with it. You, I think theorized, maybe you were talking about data, that most people would prefer their car to be equipped with an AI that tilted in favor of their passenger, but they wanted
all other cars tilted in the favor of the bus. I don't even know if it was their passenger, but themselves. People are so-called utilitarian, like, let's save the most lives, as long as the lives involved aren't their own. I don't actually know if a passenger is one of the control conditions people have done in these studies yet or not, it's an interesting question, and it might depend, like, is the passenger my brother or is the passenger my child or is it somebody I'm giving a lift in the nubar? Right. You know, probably people are a little bit less concerned about, you know, the hitchhiker or their lift passenger than they are about themselves. So if you take it out themselves in any relations and think about it abstractly, they're like, you know, save the school bus, but, you know, the closer you get to home or you're like, hey, it's my car, I paid for it. So isn't that a marketing question that fits into all of this? I mean, some of it is, and eventually we might have, you know, real discussions about this and, like, what the laws should be over it.
I mean, it's already the case that people who build these systems have to think about it some. They have to think about a cost in hitting another vehicle and so forth. They're not going to be at the level where they can really suss out how many, or I don't think anybody's to the level where they're guessing the likely number of passengers in that other vehicle. They think about it a little bit, but I think one of the constraints right now actually is you have to be able to run the computer that does all of this off a battery that can stick in the car and have the car, you know, be able to go more than a certain number of miles. And it takes a lot of energy driving the computers, making the computers operate such that you can only do certain things. There's like a big race to get enough computation done per second or per millisecond or whatever, given the size of the computer that you can actually fit. And that stuff will get better at time and computers will get smaller and more efficient and so forth. And you'll be able to do richer analyses than you can currently do. As you're saying that, it strikes me that the obvious way somebody is going to point
to is, well, we'll put all that computation in the cloud. Yeah, right now the bandwidth communicating in the cloud is way too slow. I mean, you know how when you download the news page and sometimes it hangs, that's the ping time and you don't want that in your driverless car. Really don't know. You don't want to hit the cloud and be like, oh, sorry, the reason your son died is because we had a 30 millisecond delay, you know, I don't know, the internet was just not working that day. We're really sorry. Well, that raises the question of are insurance companies sitting at the table as all this stuff is being hammered out because who's, who's insured, you know, when the driver is out of the picture, then liability, liability goes to the manufacturer. Well, it may go to the manufacturer. There's been discussion of this. I don't think anything's decided. I think there's kind of like a philosophical angle on this, there's a legal precedent angle on this. There's also a political one that I think is going to depend on who dies in driverless
cars and what are the circumstances. So, so far one person in the United States, I think there's another in China, but I haven't got full confirmation of this, have died in driverless car related accidents. Not very many. The person who died was apparently watching Harry Potter in the back seat, he owned a Tesla of people who were like, okay, that rich kid was kind of not using the cars directed and people were not sympathetic. However, you might have a situation in which driverless car does cause a less to go off of a bridge, in which case there would be lots of discussion in Congress about whether we should ban this and so forth. So eventually they're going to be fatalities. There's no way to build absolutely perfect driverless cars, any more than, you know, you can build perfect driverless driving humans. So they're going to be accidents. Some of those accidents are going to be really peculiar from the perspective of humanity as was the one the other day, mercifully nobody died, but when when the Tesla drove into the fire truck, like humans look at that and they're like, why would you do that WTF? And you know, the machines reasoned differently than people.
And some of the things they do are going to seem so odd that it might cause any number of, I mean, it's a sort of wild card effect, it might cause any number of things to happen in Congress or whatever, I can't really predict all of that. So that will enter in along with kind of philosophical questions and traditional legal considerations about responsibility. I don't know how it's all going to turn out. My own feeling is driverless cars are good thing, not as they exist now, but as they should exist, because humans are lousy drivers. They get distracted by their cell phones, they get sleepy, some of them drink. And it is certainly possible and principled to build something that's a lot safer. I think 100 years from now people look back at our time and say, you know, United States alone, 40,000 people died in the rose, like that was that savagery. Why did they even allow cars, given how bad that was? So we will reach the point where the machines are better and they're significantly better, there's no question about it. And less a kind of legal slash political slash dramatic incident deters us from that. Okay.
One more kind of bigger, bigger picture question and I know, let you, let you go back to your serious work. The human brain is more than a computational, cogutational organ, it exists in a sea of chemicals. It's an electrochemical machine and the AI brains are purely electric, there's no chemical element in them. And the chemicals, the brain is swimming in are hormones and other modifiers of brain chemistry that carry, as in the case of the instinct, flight fight and shock, modifiers of the brain's ability or availability for computation or cogutation at a particular moment for a particular reason. And there's some kind of moderating device force in the brain that is mediating, sorry,
not moderating, but mediating between the cogutational elements and these chemical messages. I have no idea how that happens, but at some point and in some kinds of personalities, the emotions override the cogutation and other kinds of personalities, the cogutation overrides the emotions. But fear is a good reason for not doing some things. You could sit there and think, what would be a great idea to, oh, there are these soft targets and these would be great targets for some sort of mischief and then there's a part of you that's afraid of being caught and a part of you that's afraid of being in a bad person and so you toss that aside as sort of a dangerous fancy and go on about your day. There's no such mediating force in the artificial intelligence world is there in those
brains, so-called. No, I mean, look, you can build certain things in, there's two things I want to say there. One is I'm not sure how important that difference is for AI and the other is I think with respect to neuroscience, it's profound. So with respect to AI, you can build an emergency system. So people in their driverless cars think about like, how do I make sure even if the kind of cognitive part of this system doesn't know what's going on, if the radar or something like that or the lighter tells me there's something in the way, I'll try to have an emergency stop. Turns out that's self-complicated because someone might plow into you and whatever. People think about these kinds of things. You can have the kind of emergency override systems. You can have sort of state dependence in the way that you're describing in a computer. I don't see any principled reason why we have to do it in silico in the same way that we do in carbon-based creatures.
On the other hand, the stuff you were talking about actually very much maps onto a debate that we've been having in neuroscience, which is another field I work in, about why is it so hard for us even to understand something as simple as a worm? So for the worm, we know that the wiring diagram is, and we've known for almost 30 years or something like that longer than 30 years. So it was 302 neurons in the Hermaphrodite version of the nematode worm, and somebody wrote out the wiring diagram in the 70s or 80s, and I'm struggling right now to think of exactly when, and you would think, okay, well, then let's just go build a computer model of a worm, and people have not been able to do that successfully, and it's not like worms are so complicated, in their behavioral repertoire, and they're not like flies with bridge mating dances. Nobody's been able to do it, and one of the reasons, which Corey Bargman has pointed out in Eve Mart, or Corey's now running the Chan Zuckerberg Institute that's trying to wipe out disease, one of the things that she's pointed out in her scientific work is
that it's about neuromodulators, which is basically what you're talking about. So even a worm actually has states like it's hungry, it's tired, and so forth, and that flips how the entire system operates, and we kind of know that now, partly through Corey's work and Eve Mart, but we have not fully assimilated that into our model of how brains work, and so all that kind of stuff that you're calling modulators and chemicals and stuff like that are pretty important to figuring out what the computations of the brain are. I'm not sure that we should say they aren't computations, but they're different kind of computation, they get integrated, so that they kind of flip the state of what the circuit is doing in deep ways that we don't yet understand. One final, just technical question about driverless cars. As I think I've read in one of your pieces, they have at least, maybe it wasn't one of yours, but they have at least three or four different sensory systems that are being integrated in real time, LiDAR, radar, a couple more, is that right?
Cameras. Cameras. There's actually variation in that across the industry. I don't think that's something you read in what I wrote, but it's true, so not every system has LiDAR, the early Teslas that had some form of quote autopilot, which is misnames since it's not really a full-time pilot. Used only cameras and didn't have LiDAR at all, and they produced good results in some context and not in others. Clearly you would like to have what people would now, and that technical jargon, call it full sensor package. You're going to do better if you've got LiDAR, maybe some radar, some cameras, and it's interesting to ask why people don't need LiDars and radars and stuff like that, and still manage to drive just with the equivalent of the cameras. The AI systems are so dumb, they need this extra information because they can't do it from the optical information or from the visual information alone. But in terms of the computation load you were talking about on the battery, I worked a lot in motion capture recently where there was the need to integrate several different
sensory inputs in real time in order to get the hands of the face and the body working together, and it took a lot of juice, so it took a lot of power to make that happen. It sounds like it's an easy thing to integrate those three sensory systems, but it's not, is it? It's not. I mean, certainly a power consideration, so if you're familiar at all with Microsoft Connect where it's taking depth readings of your body, it's doing a lot of processing in real time to generate what's called a point cloud, and you need to do essentially something like that, and then it's the same thing in motion capture. There's some kind of complex mathematical problems that need to be solved that take a lot of power. It's also the case that in the real world you often have incomplete information, and the sensors aren't registering exactly the same scene, and so you have to do some complex inference about what is the alignment between these different things that I see?
So you have to do a version of that just with your two eyes, right? Your two eyes see slightly different scenes, you're persuading yourself by closing one of the eyes and comparing. So you have to do a complicated version of that, and that's not trivial. Not trivial is this whole conversation, Gary. I can't thank you enough for sharing with us today a very insightful and knowledgeable view of this stuff that's swirling around us. I would rather have you in my home than Alexa any old time. Thank you. Thank you so much. And now ladies and gentlemen, the apologies of the week. Dateline Los Angeles rolling stones guitarist Keith Richards has apologized for suggesting that his friend and bandmate, Mick Jagger, whose father ate children so far, now should get a vasectomy. 74-year-old Richards, hey, happy birthday, mate.
He has four children and five grandchildren, himself, called Jagger, quote, a Randi old bastard in an interview with the Wall Street Journal magazine. It's not your grandfather's Wall Street Journal, apparently. Well, it's Rupert Murdox. It's time for the SNP. You can't be a father of that age. Those poor kids, he said, Jagger. Jagger became a father for the eighth time. December 2016, when his dancer, girlfriend, well, there you go right there, gave birth to a son in New York, quote, I deeply regret the comments which I made about Mick in the Wall Street Journal, which were completely out of line. I have, of course, apologized to him in person. Richards said on Twitter, Richards and Jagger, who've written most of the stone songs over the band's five-decade career, are known for their love-hate relationship. Well, beats not calling. The last week's Facebook video lamenting gun control advocates, incited hundreds of comments and spawned dozens of letters to the editor, Garfield County, Colorado, shared Lou Valario,
as followed up this week with a more reconciling message. An installment of his video commentary, Valario apologized for some of what he admitted to be divisive language last week. But he also reiterated he believes school safety measures and mental health awareness are better solutions to school shootings than gun control. But I compartmentalized people into categories, and that distracted from the message, he said, reading from a written statement. For that, I apologize he added, he added, he went on, and asked for your indulgence as I attempt to take this conversation in a different direction, and provide professional analysis based on 31 years of law enforcement experience. Instead of pointing fingers which he felt may have distracted from last week's message, he said policy makers, schools, parents, and the community all need to be part of the solution when it comes to keeping students safe. There was no mention of liberal politicians or Hollywood elite this week, two groups he singled out last week and rallying against gun control. Those were some of the fingers he pointed, or some of the targets of his finger pointed.
Quote, hopefully we can come together as a community in a nation and develop public policy training and security to minimize the ability for evil psychopaths to commit these heinous acts on our children, said Sheriff Valario. He had no comment about good psychopaths. The apologies of the week ladies and gentlemen, a copyrighted feature of this broadcast. And now, just like that, news of the warm won't you? The award-winning news of the warm, if it makes any difference to you. It does to me, but not much. Well, here in London, once this program is originating this week, we're experiencing the frosty cold and the blinding snow of the beast from the east. The northeast of the United States is experiencing a bitterly cold northeaster. And it's above freezing at the north frigging pole.
Some would rise there until March 20th. It's normally close to the coldest time of the year. But an extraordinary and possibly historic thought swelled over the pole, according to the Washington Post. Analysis show the temperature warmed to the melting point as an enormous storm pumped an intense pulse of heat through the Greenland Sea. Temperatures may have soared as high as 35 degrees Fahrenheit at the pole, according to the U.S. Global forecast system model. There are no direct measurements of temperature at the north pole, but a climate scientist working on his PhD at UC Irvine confirmed that several independent analyses showed it was very close to freezing, more than 50 degrees above normal. Normally normal anymore, wherever you look, the warm intrusion penetrated right through the heart, pardon the expression, of the central arctic, the temperature average for the entire region north of 80 degrees latitude, spiked to a highest level, ever recorded in February, I blame February, the average temperature was more than 36 degrees above normal.
No other warm intrusions were very close to this, said the researcher, describing a data set maintained by the Danish Meteorological Institute. I'll have a Danish Meteorological Institute with my coffee, that goes back to 1958. I was taken by surprise at the researcher how expansive this warm intrusion was. 2018 is well exceeding previous years for the month of February. Such extreme warm intrusions in the Arctic were once rare, now becoming more routine research has shown. A study published last July found that since 1980, these events are becoming more frequent, longer lasting, and more intense, and cheaper too. Previously this was not comments that lead author of the study Robert Graham from the Norwegian Polar Institute, it happened four years between 1980 and 2010, but now has occurred in four out of the five last winters, and nine out of the ten doctors. Graham explained that these warming events are related to the decline of winter sea ice
in the Arctic, noting that January's ice extent was the lowest on record, as the ice is thinning and melting, it's becoming more vulnerable to these winter storms. He said the thinner the ice, it drifts more quickly and can break up into smaller pieces, as strong winds from the south push the ice further north into the central Arctic, exposing the open water and releasing heat to the atmosphere from the ocean. Scientists were shocked in recent days to discover open water north of Greenland, an area normally covered by old, very thick ice, much like me. This has me more worried than the warm temps in the Arctic right now, tweeted Mike McFarron and ice sheet specialist at the University of Colorado, such warm water appears to have an effect on air temperatures at the north tip of Greenland, about 400 miles south of the north pole, the weather station, Cape Morris, just as logged a record breaking 61 hours above freezing so far this year, the previous record dating to 1980 was 16 hours, why they
just reversed the numerals there through the end of April in 2011, at one point at that north tip of Greenland, the temperature was high as 43 degrees Fahrenheit, I'll see you up there, bring your swimsuit, Kent Moore, a professor of atmospheric physics at the University of Toronto who published a study a couple years ago linking the loss of sea ice to these warm events in the Arctic, says number of factors can be contributing to the latest episode, recent storms have tracked more toward the north pole through the Greenland Sea, drawing heat directly north from lower latitudes rather than via a more circuitous route over the barrens sea, it's the battle of the seas, he also said ocean temperatures in the Greenland Sea are warmer than normal, the warmth we're seeing in Greenland Sea is definitely enhancing the warm events we're seeing, he said, I'm surprised how warm
it is, but I'm not sure why, join the club babe, news of the warm ladies and gentlemen I'm going to copyright a feature of this broadcast. Tip of the show, shout out to Gary Marcus for contributing, so my lead to today's broadcast also to Paul Herdebees at Margarita makes in Santa Monica and Justin Foy at Radio Woodstock in Woodstock for engineering the interview, next is always to Pam Hollestead and to Jenny Lawson, I'll see you, I won't see you, I really won't see you on Twitter at the Harry
Shire and you can email me and I really will read it at harryshire.com, there's an email address right there, imagine that, the show comes to you from century progress productions and originates through the facilities of WWW and New Orleans flagship station of the Change Is Easy Radio Network, so long from someplace really cold.
- Series
- Le Show
- Episode
- 2018-03-04
- Producing Organization
- Century of Progress Productions
- Contributing Organization
- Century of Progress Productions (Santa Monica, California)
- AAPB ID
- cpb-aacip-45314b493b1
If you have more information about this item than what is given here, or if you have concerns about this record, we want to know! Contact us, indicating the AAPB ID (cpb-aacip-45314b493b1).
- Description
- Segment Description
- 00:00 | 00:07 | Interview with Gary Marcus, NYU Professor of Psychology and Neural Science, on artificial intelligence | 49:32 | The Apologies of the Week : Keith Richards | 52:26 | News of the Warm | 57:21 | 'Backward Step' by Nicholas Payton /Close |
- Broadcast Date
- 2018-03-04
- Asset type
- Episode
- Media type
- Sound
- Duration
- 00:59:05.338
- Credits
-
-
Host: Shearer, Harry
Producing Organization: Century of Progress Productions
Writer: Shearer, Harry
- AAPB Contributor Holdings
-
Century of Progress Productions
Identifier: cpb-aacip-dce9ae7a53f (Filename)
Format: Zip drive
If you have a copy of this asset and would like us to add it to our catalog, please contact us.
- Citations
- Chicago: “Le Show; 2018-03-04,” 2018-03-04, Century of Progress Productions, American Archive of Public Broadcasting (GBH and the Library of Congress), Boston, MA and Washington, DC, accessed February 23, 2025, http://americanarchive.org/catalog/cpb-aacip-45314b493b1.
- MLA: “Le Show; 2018-03-04.” 2018-03-04. Century of Progress Productions, American Archive of Public Broadcasting (GBH and the Library of Congress), Boston, MA and Washington, DC. Web. February 23, 2025. <http://americanarchive.org/catalog/cpb-aacip-45314b493b1>.
- APA: Le Show; 2018-03-04. Boston, MA: Century of Progress Productions, American Archive of Public Broadcasting (GBH and the Library of Congress), Boston, MA and Washington, DC. Retrieved from http://americanarchive.org/catalog/cpb-aacip-45314b493b1